自定义的Hadoop Key数据类型
Hadoop MapReduce key类型的实例应该具有相互比较(用于排序)的能力。 要能被用作MapReduce计算中的key类型,一个Hadoop Writable数据类型应该实现org.apache.hadoop.io.WritableComparable<T> 接口。 该WritableComparable接口继承自org.apache.hadoop.io.Writable接口,并增加了compareTo()方法来执行比较。
案例描述
下面我们为HTTP服务器日志项实现一个Hadoop Writable数据类型。 这里我们假定一个日志项由五部分组成:request host、timestamp、request URL、response size和HTTP状态码。如下所示:
192.168.0.2 - - [01/Jul/1995:00:00:01 -0400] "GET /history/apollo/HTTP/1.0" 200 6245
其中:
- 199.72.81.55 客户端用户的ip
- 01/Jul/1995:00:00:01 -0400 访问的时间
- GET HTTP方法,GET或POST
- /history/apollo/ 客户请求的URL
- 200 响应码 404
- 6245 响应内容的大小
要求:实现一个自定义的Hadoop Writable数据类型用于HTTP服务器日志项。
思路
如果某个数据类型要被用作一个MapReduce计算的key数据类型,那么该数据类型必须实现org.apache.hadoop.io.WritableComparable接口。 修改之前的LogWritable数据类型,以实现WritableComparable接口,使用request hostname和timestamp来比较。
一、创建Java Maven项目
Maven依赖:
<?xml version="1.0" encoding="UTF-8"?> <project xmlns="http://maven.apache.org/POM/4.0.0" xmlns:xsi="http://www.w3.org/2001/XMLSchema-instance" xsi:schemaLocation="http://maven.apache.org/POM/4.0.0 http://maven.apache.org/xsd/maven-4.0.0.xsd"> <modelVersion>4.0.0</modelVersion> <groupId>HadoopDemo</groupId> <artifactId>com.xueai8</artifactId> <version>1.0-SNAPSHOT</version> <dependencies> <!--hadoop依赖--> <dependency> <groupId>org.apache.hadoop</groupId> <artifactId>hadoop-common</artifactId> <version>3.3.1</version> </dependency> <!--hdfs文件系统依赖--> <dependency> <groupId>org.apache.hadoop</groupId> <artifactId>hadoop-hdfs</artifactId> <version>3.3.1</version> </dependency> <!--MapReduce相关的依赖--> <dependency> <groupId>org.apache.hadoop</groupId> <artifactId>hadoop-mapreduce-client-core</artifactId> <version>3.3.1</version> </dependency> <dependency> <groupId>org.apache.hadoop</groupId> <artifactId>hadoop-mapreduce-client-jobclient</artifactId> <version>3.3.1</version> </dependency> <!--junit依赖--> <dependency> <groupId>junit</groupId> <artifactId>junit</artifactId> <version>4.12</version> <scope>test</scope> </dependency> </dependencies> <build> <plugins> <!--编译器插件用于编译拓扑--> <plugin> <groupId>org.apache.maven.plugins</groupId> <!--指定maven编译的jdk版本和字符集,如果不指定,maven3默认用jdk 1.5 maven2默认用jdk1.3--> <artifactId>maven-compiler-plugin</artifactId> <configuration> <source>1.8</source> <!-- 源代码使用的JDK版本 --> <target>1.8</target> <!-- 需要生成的目标class文件的编译版本 --> <encoding>UTF-8</encoding><!-- 字符集编码 --> </configuration> </plugin> </plugins> </build> </project>
首先,编写一个实现了org.apache.hadoop.io.WritableComparable接口的LogWritable类。
LogWritable.java:
package com.xueai8.logkey; import java.io.DataInput; import java.io.DataOutput; import java.io.IOException; import org.apache.hadoop.io.IntWritable; import org.apache.hadoop.io.Text; import org.apache.hadoop.io.WritableComparable; // 自定义LogWritable类 public class LogWritable implements WritableComparable<LogWritable> { private Text userIP, timestamp, request; private IntWritable responseSize, status; // 必须要有无参构造器 public LogWritable() { this.userIP = new Text(); this.timestamp = new Text(); this.request = new Text(); this.responseSize = new IntWritable(); this.status = new IntWritable(); } public void set (String userIP, String timestamp, String request, int bytes, int status){ this.userIP.set(userIP); this.timestamp.set(timestamp); this.request.set(request); this.responseSize.set(bytes); this.status.set(status); } // 反序列化操作 @Override public void readFields(DataInput in) throws IOException { userIP.readFields(in); timestamp.readFields(in); request.readFields(in); responseSize.readFields(in); status.readFields(in); } // 序列化操作 @Override public void write(DataOutput out) throws IOException { userIP.write(out); timestamp.write(out); request.write(out); responseSize.write(out); status.write(out); } /*** 可比较的,按ip和timestamp ***/ @Override public int compareTo(LogWritable o) { if (userIP.compareTo(o.userIP) == 0) { return timestamp.compareTo(o.timestamp); } else return userIP.compareTo(o.userIP); } public int hashCode(){ return userIP.hashCode(); } public Text getUserIP() { return userIP; } public Text getTimestamp() { return timestamp; } public Text getRequest() { return request; } public IntWritable getResponseSize() { return responseSize; } public IntWritable getStatus() { return status; } }
说明:
在WritableComparable接口中,除了Writable接口的readFields()和write()方法外,还引入 了compareTo()方法。 如果这个对象小于、等于或大于另一个进行比较的对象时,compareTo()方法应该返回一个负整数、零或一个正整数。 在这个LogWritable实现中,如果用于的ip地址和timestamps都相同的话,我们认为这两个对象是相等的。 如果这两个对象不相等,则进行排序,首先基于用户ip地址,然后基于timestamps:
public int compareTo(LogWritable o) { if (userIP.compareTo(o.userIP)==0){ return (timestamp.compareTo(o.timestamp)); }else return (userIP.compareTo(o.userIP); }
Hadoop使用HashPartitioner作为默认的partitioner实现来计算中间数据分发到的Reducer。 HashPartitioner要求key对象的hashCode()方法满足如下两个属性:
- 跨不同的JVM实例提供相同的hash值
- 提供hash值的均匀分布
因此,必须实现一个稳定的hashCode()方法,用于满足上面提到的两个要求的自定义的Hadoop key类型。 在该LogWritable实现中,我们使用request hostname/IP地址的hash码作为该LogWriter实例的hash码。 这确保中间的LogWritable数据将基于request hostaname/IP地址被分区。
public int hashCode(){ return userIP.hashCode(); }
在MapReduce计算中,既可以使用LogWritable类型作为一个key类型,也可以使用LogWritable类型作为一个value类型。 在下面的示例中,我们使用该LogWritable类型作为Map输出的key类型。
LogMapper.java:
package com.xueai8.logkey; import org.apache.hadoop.io.IntWritable; import org.apache.hadoop.io.LongWritable; import org.apache.hadoop.io.Text; import org.apache.hadoop.mapreduce.Mapper; import java.io.IOException; import java.util.regex.Matcher; import java.util.regex.Pattern; /** * * 使用 LogWritable 类型作为Map输出的key类型 * 实现自动去重(同一IP同一时间的访问为重复数据) */ public class LogMapper extends Mapper<LongWritable,Text, LogWritable, IntWritable>{ private final LogWritable outKey = new LogWritable(); private final IntWritable outValue = new IntWritable(0); @Override protected void map(LongWritable key, Text value, Context context) throws IOException, InterruptedException { // 提取相应字段的正则表达式 String regexp = "^(\\S+) (\\S+) (\\S+) \\[([\\w:/]+\\s[+\\-]\\d{4})\\] \"(.+?)\" (\\d{3}) (\\d+)"; Pattern pattern = Pattern.compile(regexp); Matcher matcher = pattern.matcher(value.toString()); if(!matcher.matches()) { System.out.println("不是一个有效的日志记录"); return; } // 提取相应的字段 String ip = matcher.group(1); String timestamp = matcher.group(4); String url = matcher.group(5); int status = Integer.parseInt(matcher.group(6)); int responseSize = Integer.parseInt(matcher.group(7)); // LogWritable为 value outKey.set(ip, timestamp, url, status, responseSize); outValue.set(responseSize); context.write(outKey, outValue); // 写出 } }
在下面的示例中,我们使用该LogWritable类型作为Reduce输入的key类型。
LogReducer.java:
这里我们统计每个IP的下载量。
package com.xueai8.logkey; import org.apache.hadoop.io.IntWritable; import org.apache.hadoop.io.Text; import org.apache.hadoop.mapreduce.Reducer; import java.io.IOException; /** * * 计算每个IP某一时刻的下载量 */ public class LogReducer extends Reducer<LogWritable, IntWritable, Text, IntWritable> { private final Text outKey = new Text(); private final IntWritable outValue = new IntWritable(0); @Override protected void reduce(LogWritable key,Iterable<IntWritable> values, Context context) throws IOException, InterruptedException { int total = 0; for(IntWritable value : values) { total += value.get(); } outValue.set(total); outKey.set(key.getUserIP() + "_" + key.getTimestamp()); context.write(outKey, outValue); // 写出 } }
LogDriver.java:
作为输入,这个应用程序可以接收任何文本文件。可直接从IDE运行LogDriver类并传递input和output作为参数。
package com.xueai8.logkey; import java.io.IOException; import org.apache.hadoop.conf.Configuration; import org.apache.hadoop.fs.Path; import org.apache.hadoop.io.IntWritable; import org.apache.hadoop.io.Text; import org.apache.hadoop.mapreduce.Job; import org.apache.hadoop.mapreduce.lib.input.FileInputFormat; import org.apache.hadoop.mapreduce.lib.output.FileOutputFormat; public class LogDriver { public static void main(String[] args) throws IllegalStateException, IllegalArgumentException, ClassNotFoundException, IOException, InterruptedException { if(args.length < 2) { System.out.println("用法: LogDriver <input> <output>"); System.exit(1); } Configuration conf = new Configuration(); Job job = Job.getInstance(conf,"日志分析"); job.setJarByClass(LogDriver.class); // set mapper job.setMapperClass(LogMapper.class); job.setMapOutputKeyClass(LogWritable.class); job.setMapOutputValueClass(IntWritable.class); // set reducer job.setReducerClass(LogReducer.class); job.setOutputKeyClass(Text.class); job.setOutputValueClass(IntWritable.class); // 设置输入路径 FileInputFormat.setInputPaths(job, new Path(args[0])); FileOutputFormat.setOutputPath(job, new Path(args[1])); // 提交作业 System.exit(job.waitForCompletion(true) ? 0 : 1); } }
二、配置log4j
在src/main/resources目录下新增log4j的配置文件log4j.properties,内容如下:
log4j.rootLogger = info,stdout ### 输出信息到控制抬 ### log4j.appender.stdout = org.apache.log4j.ConsoleAppender log4j.appender.stdout.Target = System.out log4j.appender.stdout.layout = org.apache.log4j.PatternLayout log4j.appender.stdout.layout.ConversionPattern = [%-5p] %d{yyyy-MM-dd HH:mm:ss,SSS} method:%l%n%m%n
三、项目打包
打开IDEA下方的终端窗口terminal,执行"mvn clean package"打包命令,如下图所示:
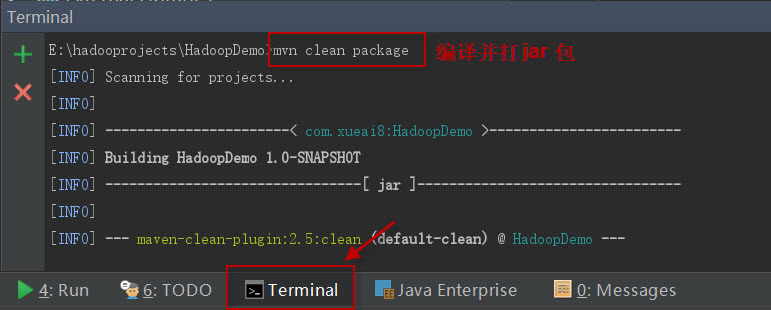
如果一切正常,会提示打jar包成功。如下图所示:
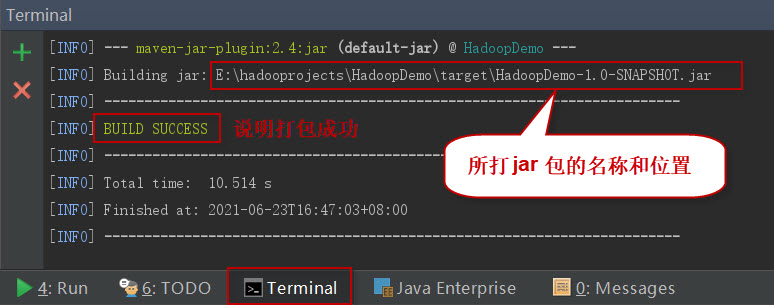
这时查看项目结构,会看到多了一个target目录,打好的jar包就位于此目录下。如下图所示:
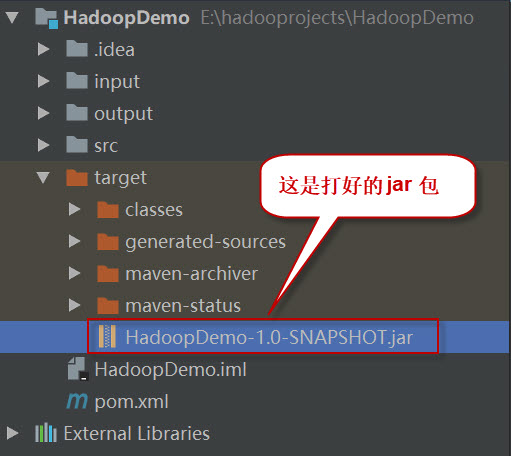
四、项目部署
请按以下步骤执行。
1、启动HDFS集群和YARN集群。在Linux终端窗口中,执行如下的脚本:
$ start-dfs.sh $ start-yarn.sh
查看进程是否启动,集群运行是否正常。在Linux终端窗口中,执行如下的命令:
$ jps
这时应该能看到有如下5个进程正在运行,说明集群运行正常:
5542 NodeManager 5191 SecondaryNameNode 4857 NameNode 5418 ResourceManager 4975 DataNode
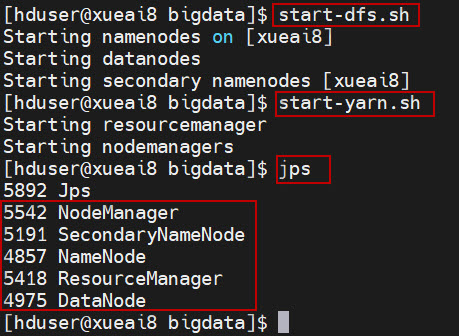
2、将日志数据文件log_sample.txt上传到HDFS的/data/mr/目录下。
$ hdfs dfs -mkdir -p /data/mr $ hdfs dfs -put log_sample.txt /data/mr/ $ hdfs dfs -ls /data/mr/
3、提交作业到Hadoop集群上运行。(如果jar包在Windows下,请先拷贝到Linux中。)
在终端窗口中,执行如下的作业提交命令:
$ hadoop jar HadoopDemo-1.0-SNAPSHOT.jar com.xueai8.logkey.LogDriver /data/mr /data/mr-output
4、查看输出结果。
在终端窗口中,执行如下的HDFS命令,查看输出结果:
$ hdfs dfs -ls /data/mr-output $ hdfs dfs -cat /data/mr-output/part-r-00000
可以看到最后的统计结果如下:
129.94.144.152_01/Jul/1995:00:00:13 -0400 7074 129.94.144.152_01/Jul/1995:00:00:17 -0400 0 199.120.110.21_01/Jul/1995:00:00:09 -0400 4085 199.120.110.21_01/Jul/1995:00:00:11 -0400 4179 199.120.110.21_01/Jul/1995:00:00:17 -0400 1713 199.72.81.55_01/Jul/1995:00:00:01 -0400 6245 199.72.81.55_01/Jul/1995:00:00:59 -0400 1382 199.72.81.55_01/Jul/1995:00:01:43 -0400 7074 199.72.81.55_01/Jul/1995:00:01:46 -0400 5866 199.72.81.55_01/Jul/1995:00:01:50 -0400 363 199.72.81.55_01/Jul/1995:00:01:51 -0400 234 199.72.81.55_01/Jul/1995:00:01:52 -0400 669 205.189.154.54_01/Jul/1995:00:00:24 -0400 3985 205.189.154.54_01/Jul/1995:00:00:29 -0400 40310 205.189.154.54_01/Jul/1995:00:00:40 -0400 786 205.189.154.54_01/Jul/1995:00:00:41 -0400 1204 205.189.154.54_01/Jul/1995:00:01:06 -0400 110 205.189.154.54_01/Jul/1995:00:01:08 -0400 7634 205.189.154.54_01/Jul/1995:00:01:19 -0400 1224 205.212.115.106_01/Jul/1995:00:00:12 -0400 3985 205.212.115.106_01/Jul/1995:00:01:13 -0400 7634 alyssa.prodigy.com_01/Jul/1995:00:00:33 -0400 12054 burger.letters.com_01/Jul/1995:00:00:11 -0400 0 burger.letters.com_01/Jul/1995:00:00:12 -0400 0 d104.aa.net_01/Jul/1995:00:00:13 -0400 3985 d104.aa.net_01/Jul/1995:00:00:15 -0400 42300 dave.dev1.ihub.com_01/Jul/1995:00:01:55 -0400 3985 dave.dev1.ihub.com_01/Jul/1995:00:01:58 -0400 786 dave.dev1.ihub.com_01/Jul/1995:02:01:58 -0400 40310 dave.dev1.ihub.com_01/Jul/1995:03:01:58 -0400 1204 dd14-012.compuserve.com_01/Jul/1995:00:01:05 -0400 42732 dial22.lloyd.com_01/Jul/1995:00:00:37 -0400 61716 gater3.sematech.org_01/Jul/1995:00:01:50 -0400 40310 gater3.sematech.org_01/Jul/1995:00:01:52 -0400 1204 gater4.sematech.org_01/Jul/1995:00:01:46 -0400 3985 gater4.sematech.org_01/Jul/1995:00:01:52 -0400 786 gayle-gaston.tenet.edu_01/Jul/1995:00:00:50 -0400 12040 ix-or10-06.ix.netcom.com_01/Jul/1995:00:01:52 -0400 5998 ix-or10-06.ix.netcom.com_01/Jul/1995:00:01:57 -0400 4151 ix-orl2-01.ix.netcom.com_01/Jul/1995:00:00:41 -0400 3985 ix-orl2-01.ix.netcom.com_01/Jul/1995:00:00:44 -0400 40310 ix-orl2-01.ix.netcom.com_01/Jul/1995:00:01:18 -0400 1204 link097.txdirect.net_01/Jul/1995:00:01:26 -0400 8677 link097.txdirect.net_01/Jul/1995:00:01:27 -0400 11853 link097.txdirect.net_01/Jul/1995:00:01:31 -0400 1990 link097.txdirect.net_01/Jul/1995:00:01:44 -0400 4377 link097.txdirect.net_01/Jul/1995:00:01:45 -0400 4179 link097.txdirect.net_01/Jul/1995:00:01:47 -0400 1713 link097.txdirect.net_01/Jul/1995:00:01:55 -0400 6922 link097.txdirect.net_01/Jul/1995:00:01:56 -0400 11417 net-1-141.eden.com_01/Jul/1995:00:00:19 -0400 34029 netport-27.iu.net_01/Jul/1995:00:01:57 -0400 7074 onyx.southwind.net_01/Jul/1995:00:01:34 -0400 3985 onyx.southwind.net_01/Jul/1995:00:01:35 -0400 40310 onyx.southwind.net_01/Jul/1995:00:01:39 -0400 0 piweba3y.prodigy.com_01/Jul/1995:00:00:54 -0400 12054 piweba3y.prodigy.com_01/Jul/1995:00:01:14 -0400 55666 pm13.j51.com_01/Jul/1995:01:01:58 -0400 305722 port26.annex2.nwlink.com_01/Jul/1995:00:01:02 -0400 9867 port26.annex2.nwlink.com_01/Jul/1995:00:01:04 -0400 31073 port26.annex2.nwlink.com_01/Jul/1995:00:01:17 -0400 13372 port26.annex2.nwlink.com_01/Jul/1995:00:01:27 -0400 1567 port26.annex2.nwlink.com_01/Jul/1995:00:01:32 -0400 903 ppp-mia-30.shadow.net_01/Jul/1995:00:00:27 -0400 7074 ppp-mia-30.shadow.net_01/Jul/1995:00:00:35 -0400 5866 ppp-mia-30.shadow.net_01/Jul/1995:00:00:41 -0400 1383 ppp-mia-30.shadow.net_01/Jul/1995:00:00:43 -0400 669 ppp-nyc-3-1.ios.com_01/Jul/1995:00:00:59 -0400 77163 ppp-nyc-3-1.ios.com_01/Jul/1995:00:01:49 -0400 52491 ppptky391.asahi-net.or.jp_01/Jul/1995:00:00:18 -0400 3977 ppptky391.asahi-net.or.jp_01/Jul/1995:00:00:19 -0400 11473 remote27.compusmart.ab.ca_01/Jul/1995:00:01:14 -0400 12054 remote27.compusmart.ab.ca_01/Jul/1995:00:01:27 -0400 3985 remote27.compusmart.ab.ca_01/Jul/1995:00:01:53 -0400 110 remote27.compusmart.ab.ca_01/Jul/1995:00:01:55 -0400 7634 scheyer.clark.net_01/Jul/1995:00:00:58 -0400 49152 slip1.yab.com_01/Jul/1995:00:01:26 -0400 6168 slip1.yab.com_01/Jul/1995:00:01:29 -0400 16991 smyth-pc.moorecap.com_01/Jul/1995:00:00:38 -0400 101267 smyth-pc.moorecap.com_01/Jul/1995:00:01:19 -0400 18149 smyth-pc.moorecap.com_01/Jul/1995:00:01:24 -0400 2261 unicomp6.unicomp.net_01/Jul/1995:00:00:06 -0400 3985 unicomp6.unicomp.net_01/Jul/1995:00:00:14 -0400 42300 unicomp6.unicomp.net_01/Jul/1995:00:01:41 -0400 3214 waters-gw.starway.net.au_01/Jul/1995:00:00:25 -0400 6723 www-a1.proxy.aol.com_01/Jul/1995:00:01:09 -0400 3985 www-b4.proxy.aol.com_01/Jul/1995:00:01:21 -0400 70712